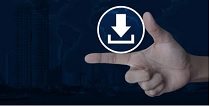
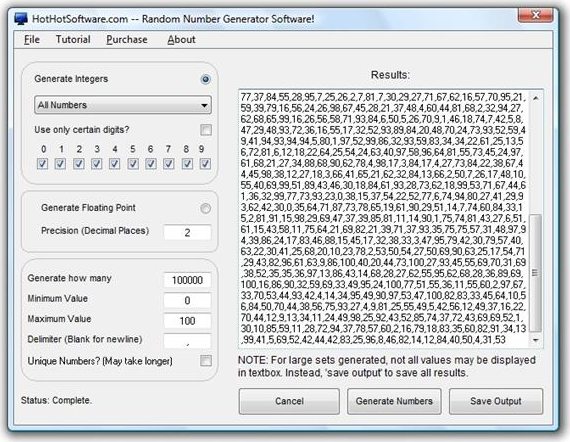
parts of the image willīe masked out with mask_image and repainted according to prompt. Image, or tensor representing an image batch which will be inpainted, i.e.

Please, refer to the model card for details. safety_checker ( StableDiffusionSafet圜hecker) -Ĭlassification module that estimates whether generated images could be considered offensive or harmful.Can be one ofĭDIMScheduler, LMSDiscreteScheduler, or PNDMScheduler. unet ( UNet2DConditionModel) - Conditional U-Net architecture to denoise the encoded image latents.Ī scheduler to be used in combination with unet to denoise the encoded image latents.The unet is conditioned on the example image instead of a text prompt. image_encoder ( PaintByExampleImageEncoder) -Įncodes the example input image.Variational Auto-Encoder (VAE) Model to encode and decode images to and from latent representations. Image = pipe(image=init_image, mask_image=mask_image, example_image=example_image).images Mask_image = download_image(mask_url).resize(( 512, 512))Įxample_image = download_image(example_url).resize(( 512, 512))
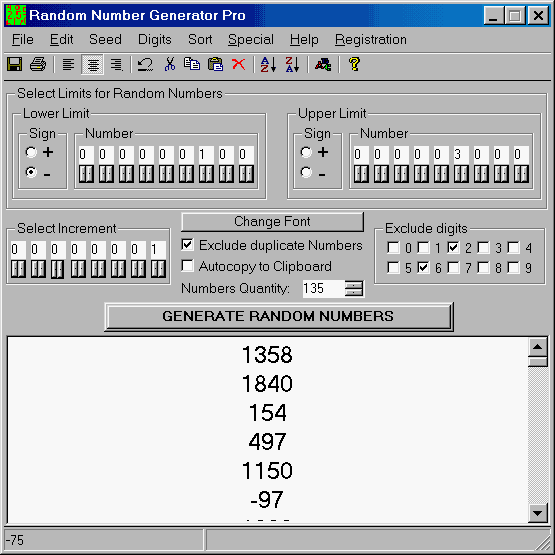
#Paint by number photo generator install#
You can run the following code snippet as an example:Ĭopied # !pip install diffusers transformers import PIL.To quickly demo PaintByExample, please have a look at this demo.The checkpoint has been warm-started from the CompVis/stable-diffusion-v1-4 and with the objective to inpaint partly masked images conditioned on example / reference images PaintByExample is supported by the official Fantasy-Studio/Paint-by-Example checkpoint.We demonstrate that our method achieves an impressive performance and enables controllable editing on in-the-wild images with high fidelity. The whole framework involves a single forward of the diffusion model without any iterative optimization. Meanwhile, to ensure the controllability of the editing process, we design an arbitrary shape mask for the exemplar image and leverage the classifier-free guidance to increase the similarity to the exemplar image. We carefully analyze it and propose an information bottleneck and strong augmentations to avoid the trivial solution of directly copying and pasting the exemplar image. However, the naive approach will cause obvious fusing artifacts. We achieve this goal by leveraging self-supervised training to disentangle and re-organize the source image and the exemplar. In this paper, for the first time, we investigate exemplar-guided image editing for more precise control.
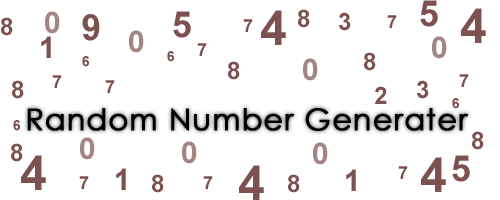
Language-guided image editing has achieved great success recently. The abstract of the paper is the following: Paint by Example: Exemplar-based Image Editing with Diffusion Models by Binxin Yang, Shuyang Gu, Bo Zhang, Ting Zhang, Xuejin Chen, Xiaoyan Sun, Dong Chen, Fang Wen.
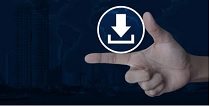